Winning the Digital Shelf On Retail Media Networks With AI
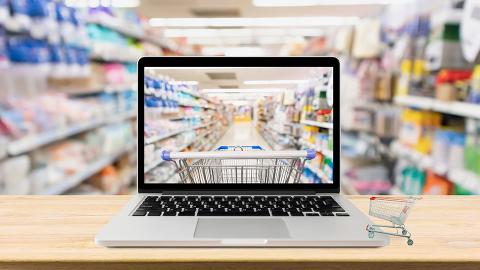
With the growing popularity of online shopping, retail media can help brands engage consumers in their purchase journey at the point of sale.A retail media network (RMN) is a retail company owned digital platform that sells ad space to marketers. Think of it like a “digital shelf” — much like how in-aisle promotions and offers can help brands get the attention of the shoppers in physical stores, retail media can help brands be even more visible to shoppers.
Retail media allow brands to bid for ad space on a retailer’s website or mobile app, and then serve up targeted ads to shoppers based on their search history, purchase history and other behavioral data. With RMNs, advertisers gain access to a retailer's first-party data, such as purchases made at the point of sale, which can help a marketer improve targeting capabilities and personalized messaging.
Additionally, accessing first-party data from retailers is a privacy-compliant substitute for cookies. Data clean rooms, which assist content platforms in maintaining the privacy of first-person user data when interacting with advertising providers, are the way forward as the world moves towards cookieless user experience. They are secure and controlled environments where companies can share, compare, and analyze sensitive user data without falling prey to any kind of compliance data breach.
In a marketplace that is replete with choices for buyers, gaining visibility among the right audience is crucial to drive brand awareness and sales, and so it's important to determine which RMNs are a priority for the brand or category vs. which ones don't fit.
Challenges of Advertising on RMNs
Inefficient optimization of campaigns: After carefully curating a campaign for digital platforms, marketers hope to optimize it in-flight to constantly improve performance and yield better results. Alongside experience, this also requires technologies such as AI and ML algorithms that can capture real-time data to automate campaign changes. Inefficient optimization plans can lead to wasted ad dollars and poor conversions.
Lack of reporting:To track the effectiveness of campaigns, marketers need reporting and metrics that can guide them to the right direction. Amazon typically provides raw data to sellers instead of granular campaign reports which can be difficult to analyze and make changes to campaigns inflight.
Inability to target relevant audiences: Brands want to continuously identify how their campaigns are performing and among which audiences are they most successful. While they can identify audience categories, they do not have access to each segment individually as Amazon restricts its customers identity through an identity link and does not disclose their phone numbers or email addresses.
3 metrics to measure marketing effectiveness
1. Return on Advertising Spend (ROAS): This is the ratio of revenue generated to advertising spend. A higher ROAS indicates better performance as it means a higher revenue generated for every dollar spent on advertising.
2. Conversion Rate (CR): This is the percentage of people who complete a desired action (such as making a purchase) after clicking on the ad. A higher CR indicates better performance as it means that the ad is effectively converting users into customers.
3. Click-Through Rate (CTR): This is the percentage of people who click on the ad after seeing it. A higher CTR indicates better performance as it means that the ad is more appealing to users.
How AI/ML can help brands improve targeting and conversions
AI allows intelligent programing of contextual ad campaigns by targeting the right consumers, thus empowering advertisers to put their dollars where it matters the most.
Modern advertisers and marketers can adapt an intelligent solution approach to execute cognitive advertising, wherein two machine learning models interact with each other and work in tandem to maximize overall ROAS.
One model aims at driving purchases and the other model works to optimize cost. Such a solution is powered by an ABC framework of display ad campaign optimization: audience, budget, and campaign settings, which overcomes the challenges of traditional advertising on RMNs.
Audience: By identifying the right target audience, brands can spend less on advertising while reaching more prospective users. For example, they can identify consumers by geolocation, purchase timing, browsing history, in-app behavior, session depth, and click tendency etc. The algorithms will then segment the audiences into multiple cohorts, and create tailored ads and post-click experiences that resonate with each group to improve the quality scores, click-through rate (CTR), and engagement on the ads.
With test-and-learn approaches, the technology analyzes best-performing offerings and matches them to relevant consumers while also taking care of ad placement — thus, driving more purchase conversions for the brand.
Budget: Machine learning enables brands to decide the right budget range to spend for every ad by trading off between full and partial investments. Machine learning algorithms help brands sift through data to identify the most successful time of the day and days in the week for highest traction on the ads. This ensures that the channels where the ads are displayed fetch the highest ROI and with the most optimized cost of leads.
It also maps the right budget with each target audience group. The budget bid for the display ads on multiple platforms are carefully calculated and compared, allowing advertisers to purchase ad impressions and placement slots based on priorities like improved clicks, shares, CPA, CPC, CPM etc. This improves the conversion rates and ensures value to advertisers by managing the bidding process for maximum profit.
Campaign Settings: Machine learning also helps target/retarget audiences based on their buying intents- strong, moderate, low and no explicit buying intent. Brands are empowered to modify and develop ad creatives for the different consumer groups.
Based on the inputs from the other model, the second model also generates other campaign configurations such as ideal bid amount, frequency of ads, keywords, ad copies, ad variants etc. This ensures the best performance of each ad for each consumer cohort. Automated campaign monitoring evaluates the campaigns to check if they are fully-optimized and recommends actions to enhance the optimization score metric.
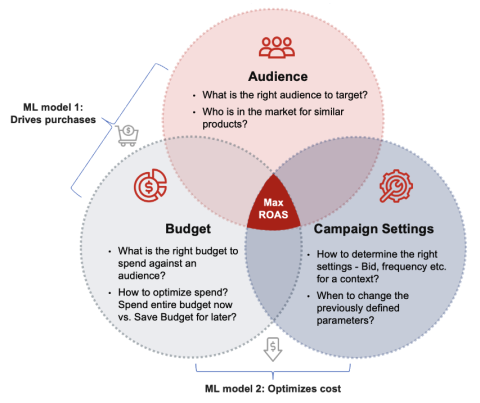
A multinational nutrition brand sought to increase spend efficiency and accelerate sales on Amazon leveraging machine learning algorithms. Using first- and third-party data along with historical campaign insights on Amazon, machine learning determined the right target audience, and optimized campaign settings like budgets, bid value. and ad frequency for each campaign, leading to a 10x increase in ROAS.
Brands can use AI and ML to optimize their display ad campaigns on retail media. Clean data rooms can help in optimizing ad campaigns on retail media networks by providing accurate and reliable data for analysis. This can lead to better targeting, improved ROI, and more effective use of ad spend.
By analyzing vast amounts of data, AI and ML can help identify the most effective targeting and messaging strategies, resulting in improved ROAS and increased revenue. For example, AI based solutions can help consumer brands select the most relevant target audience segments from Amazon that are otherwise not factored in the campaign set up process. Additionally, AI and ML can be used to automate the ad buying and placement process, allowing brands to quickly and efficiently adjust their campaigns in response to changing market conditions.
By incorporating AI and ML into their digital marketing strategies, brands can gain a competitive advantage and stay ahead of the curve in an increasingly crowded and complex digital retail landscape.
— Jayant Pandit is a Senior Director of Marketing at Sigmoid.